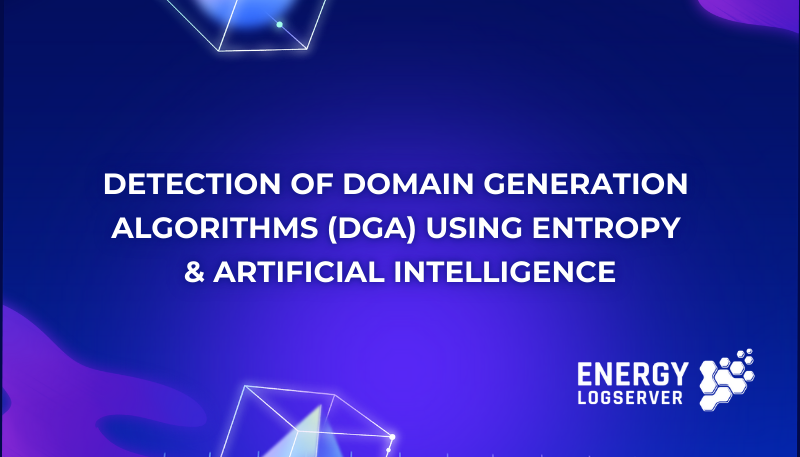
Malware often uses Domain Generation Algorithms (DGA) to avoid detection and blocking by security systems, generating dynamic domain names for connecting to command and control servers. Detecting such domains is crucial for protecting companies and networks. One effective method is analyzing the entropy of domain segments. Domains created by malware tend to exhibit higher entropy, meaning they are more random and less predictable than those created by humans, making this method useful in identifying malicious activities.
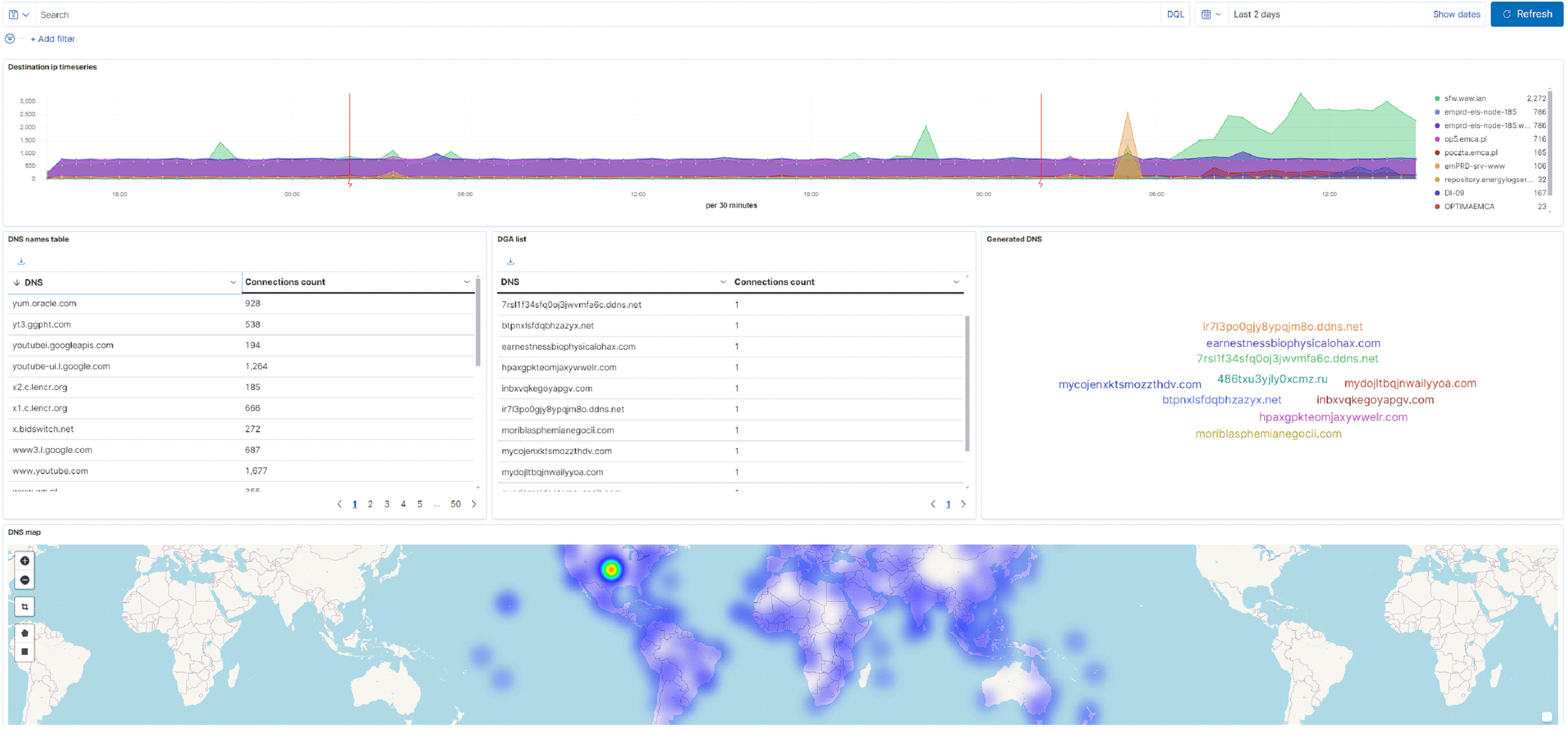
Calculating Entropy in DGA Detection
Entropy measures data unpredictability and helps identify domains generated by algorithms. Automatically generated domains often have higher randomness and entropy, making them suspicious. By analyzing entropy in domain segments, unusual patterns indicating malicious activity can be detected.
Reducing False Positives
To minimize false alarms, especially for legitimate high-entropy domains, trusted domains (whitelists) are excluded from analysis, improving accuracy.
AI and Machine Learning
In addition to entropy, AI and machine learning algorithms analyze domain complexity and patterns to enhance detection, identifying even subtle DGA-based threats.
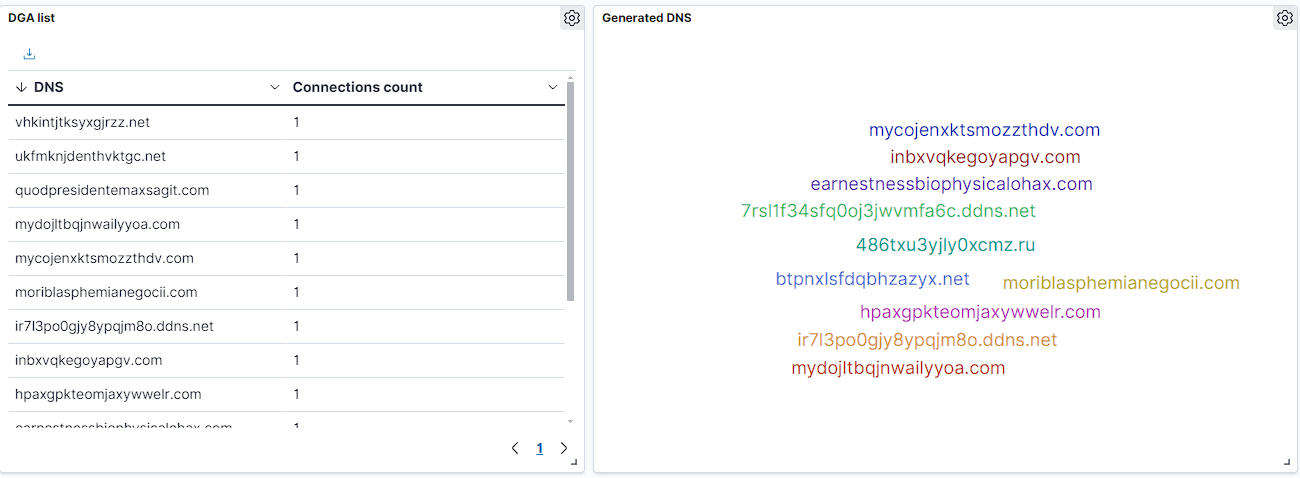
Domain Generation Algorithms (DGA) are used by malware to evade detection and blocking by dynamically creating new web addresses for communication with command and control servers. This enables malware to continue operating even if one domain is blocked. Combining traditional detection methods with AI and machine learning significantly enhances the ability to identify generated domains, leading to improved network security and stability.